Job title: Business Development Manager
Company: Celtic Careers
Job description: Business Development Manager Celtic Careers has partnered with a leading distributor of engineering materials… and solutions who is currently hiring a Business Development Manager to join their Regional Business Development team. Reporting…
Expected salary:
Location: Dublin
Job date: Sat, 13 Apr 2024 00:22:28 GMT
Apply for the job now!
What Should the Global Summit on AI Safety Try to Accomplish?
This post explores possible outcomes of the upcoming UK-hosted global summit on AI safety. It draws on ideas raised in a recent expert workshop hosted by GovAI, but is not intended as a consensus statement.
GovAI research blog posts represent the views of their authors, rather than the views of the organisation.
Summary
Following growing concern about possible extreme risks from AI, the United Kingdom recently announced plans to host the first major global summit on AI safety. The announcement sketched a high-level vision for the summit:
The summit, which will be hosted in the UK this autumn, will consider the risks of AI, including frontier systems, and discuss how they can be mitigated through internationally coordinated action. It will also provide a platform for countries to work together on further developing a shared approach to mitigate these risks.
However, more detailed aims have not yet been shared. GovAI recently convened a small expert workshop – organised without government involvement and held under the Chatham House rule – to explore possible objectives for the summit.
Attendees suggested a wide variety of valuable direct outcomes of the summit. These can could mainly be grouped into six categories:
- Producing shared commitments and consensus statements from states
- Planting the seeds for new international institutions
- Highlighting and diffusing UK AI policy initiatives
- Securing commitments from AI labs
- Increasing awareness and understanding of AI risks and governance options
- Committing participants to annual AI safety summits and further discussions
Several attendees suggested that the summit may be a unique and fleeting opportunity to ensure that global AI governance includes China. China is likely to be excluded from important discussion venues, such as the OECD and G7. China may also have only a temporary period of AI policy “plasticity” and will be more inclined to reject any global governance principles that Western states begin crafting without its input. However, one attendee disagreed that China should be invited, emphasising that Chinese participation could make the summit less productive.
The Summit Could Produce Several Valuable Outcomes
Potential valuable outcomes from the summit include:
- Producing shared commitments and consensus statements from states. Participating states could sign onto statements that establish a shared understanding of risk, influence global AI governance norms and priorities, and provide guidance to private labs. A baseline goal could be to produce a shared statement acknowledging that extreme safety risks are a global priority.
- Planting the seeds for new international institutions. States could commit to creating new international institutions (or at least further discussing possibilities). Possible functions of new institutions include housing research, developing expert consensus and shared standards, providing evaluation and auditing functions, and facilitating the creation of international agreements.
- Highlighting and diffusing UK AI policy initiatives. The UK could establish itself as the global leader in AI policy, showcase its new AI policy initiatives, and support their diffusion to other states. Initiatives that could be showcased include an information-sharing and model evaluation regime, plans for an associated licensing regime, and guidance documents to labs outlining responsible practices (e.g. regarding model release and scaling).
- Securing commitments from AI labs. Labs could make shared commitments (e.g. to best practices) that they will not by default make without public pressure or government encouragement, due to commercial incentives and legal constraints that limit coordination. This could include commitments to share further information with governments, invest a minimum portion of their resources into safety, temporarily pause development if certain model evaluation results are triggered, or adopt responsible policies regarding model scaling.
- Increasing awareness and understanding of AI risks and governance options. Events (e.g. events demonstrating risks), discussions, statements, and media coverage of the summit could increase awareness and understanding of AI safety challenges (particularly AGI safety challenges) and the need for global governance. It is useful for this awareness to be shared across government, industry, civil society, the media, and the broader public.
- Committing participants to annual AI safety summits and further discussions. Participants could agree to further meetings to maintain momentum and make progress on issues raised during the summit (e.g. the potential need for new international institutions and agreements). In addition to further iterations of the summit, new expert-led working groups (potentially hosted by the UK or the OECD) could be established.
The Summit May Be a Critical Opportunity to Ensure Global AI Governance Includes China
A number of participants suggested that this summit may be the only opportunity to include China in productive global AI governance discussions. Other important venues – including the OECD, the G7, or meetings initiated by the US – will likely exclude China by default. More inclusive institutions such as the UN may also struggle to make forward progress due to structural constraints.
This opportunity may be critical, since global AI governance will likely fail if it does not ultimately include China. If China – the world’s third major AI power – does not adopt responsible AI safety policies, then this could threaten the security of all other states. External constraints, such as export controls on advanced chips and relevant manufacturing equipment, will probably only delay China’s ability to create high-risk models.
Summit outcomes (such as those listed above) could therefore be more valuable if they include China. One participant whose research focuses on Chinese AI policy argued that China can be positively influenced by the summit.1 There is interest in AI safety in China – for instance, a number of prominent Chinese academics signed the recent CAIS statement on AI risk – and Chinese actors have been signalling an interest in participating in global AI governance efforts.
Trying to involve China later – for instance, in future iterations of the summit – may also be much less impactful than involving China now. China is more likely to adopt global frameworks if it can legitimately view itself as a co-creator of these frameworks; this will be less likely if China is not invited from the start. As China develops its own regulatory frameworks for advanced AI and makes its own independent policy statements, Chinese AI policy may also quickly lose some of the “plasticity” it has now
However, within the group, there was not universal consensus that China should be invited to the summit. One potential cost of inviting China is that it may reduce the enthusiasm of other states and non-governmental actors to participate in the summit. Inviting China may also make the summit less productive by increasing the level of disagreement and potential for discord among participants. There may also be some important discussion topics that would not be as freely explored with Chinese representatives in the room.
These concerns are partly mitigated by the likely existence of side meetings – and discussions in venues beyond the summit – which could include smaller subsets of participants. However, involving China in the summit would inevitably involve trade-offs. These trade-offs could be evaluated in part by seeking the views of other likely participants.
Conclusion
The first global summit on AI safety is an important opportunity to make progress toward managing global risks from advanced AI systems. It could directly produce several outcomes, including: (1) shared commitments and consensus statements from states, (2) the seeds of new international institutions, (3) the advertisement and diffusion of UK AI policy initiatives, (4) shared commitments from labs, (5) increased understanding of AI risks and governance options, and (6) commitments to further productive discussions.
It may also be a unique and fleeting opportunity to involve China in global AI governance. Ultimately, however, making the correct decision regarding China’s participation will require weighing the value of Chinese participation with the frictions and stakeholder management challenges issues it may bring.
The authors of this piece can be contacted at
be***********@go*******.ai
and
le**********@go********.ai
.
Business Development Manager – Dublin
Job title: Business Development Manager – Dublin
Company: Elk Recruitment
Job description: to help deliver improved service and innovation to the customer. Detailed knowledge of marketing and business development…Position: Business Development Manager Location: Dublin / Hybrid Salary: Negotiable D.O.E The Job: The Business…
Expected salary: €50000 – 60000 per year
Location: Dublin
Job date: Sat, 13 Apr 2024 03:57:27 GMT
Apply for the job now!
Proposing an International Governance Regime for Civilian AI
This post summarises a new report, “International Governance of Civilian AI: A Jurisdictional Certification Approach.” You can read the full report here.
GovAI research blog posts represent the views of their authors, rather than the views of the organisation.
Many jurisdictions have begun to develop their own approaches to regulating AI, in response to concerns that range from bias to misinformation to the possibility of existential risks from advanced AI.
However, without international coordination, these purely national and regional approaches will not be enough. Many of the risks posed by AI are inherently international, since they can produce harms that spill across borders. AI supply chains and product networks also span many countries. We therefore believe that a unified international response to the risks posed by AI will be necessary.
In recognition of this need, we have developed a proposal – outlined in a new report – for an international governance body that can certify compliance with international standards on civilian AI.1 We call this proposed body the International AI Organization (IAIO).
Our proposal for the IAIO follows a jurisdictional certification approach, modelled on the approach taken by other international bodies such as the International Civilian Aviation Organization (ICAO), the International Maritime Organization (IMO) and the Financial Action Task Force (FATF). Under this approach, the international body certifies that the regulatory regimes adopted within individual jurisdictions meet international standards. Jurisdictions that fail to receive certifications (e.g. because their regulations are too lax or they fail to enforce them) are excluded from valuable trade relationships – or otherwise suffer negative consequences.
Our report outlines the proposal in more detail and explains how it could be put into practice. We suggest that even a very partial international consensus on minimum regulatory standards – perhaps beginning with just a few major players – could be channeled into an institutional framework designed to produce increasingly widespread compliance. An initial set of participating states could establish the IAIO and – through the IAIO – arrive at a set of shared standards and a process for certifying that a state’s regulatory regime meets these standards. One of the standards would be a commitment to ban the import of goods that integrate AI systems from uncertified jurisdictions. Another standard could be a commitment to ban the export of AI inputs (such as specialised chips) to uncertified jurisdictions. The participating states’ trade policies would thereby incentivise other states to join the IAIO themselves and receive certifications.
We believe that the IAIO could help to mitigate many of AI’s potential harms, from algorithmic bias to the longer-term security threats. Our hope is that it can balance the need to prevent harmful forms of proliferation against the imperatives to spread the benefits of the technology and give voice to affected communities around the globe.
Staff IT Data Engineer at Palo Alto Networks – Santa Clara, CA, United States
Company Description
Our Mission
At Palo Alto Networks® everything starts and ends with our mission:
Being the cybersecurity partner of choice, protecting our digital way of life.
Our vision is a world where each day is safer and more secure than the one before. We are a company built on the foundation of challenging and disrupting the way things are done, and we’re looking for innovators who are as committed to shaping the future of cybersecurity as we are.
Our Approach to Work
We lead with flexibility and choice in all of our people programs. We have disrupted the traditional view that all employees have the same needs and wants. We offer personalization and offer our employees the opportunity to choose what works best for them as often as possible – from your wellbeing support to your growth and development, and beyond!
At Palo Alto Networks, we believe in the power of collaboration and value in-person interactions. This is why our employees generally work from the office three days per week, leaving two days for choice and flexibility to work where you feel most effective. This setup fosters casual conversations, problem-solving, and trusted relationships. While details may evolve, our goal is to create an environment where innovation thrives, with office-based teams coming together three days a week to collaborate and thrive, together!
Job Description
Your Career
The Palo Alto Networks IT department is now a full-fledged engineering organization. Equipped with a full team of Data Engineers, Data Scientists, and next-generation Data Visualization technologies. Together we are building the ‘Predictive Enterprise’, an infrastructure that will allow Palo Alto Networks the ability to make the best and fastest business decisions based on all data available. The growth of this team includes the addition of a Data Engineer who is tasked with crafting and implementing integrations using state-of-the-art Cloud technologies.
Your Impact
- As a Data Engineer, you’ll play a crucial role in our Data & Analytics team, tasked with crafting and implementing integrations using state-of-the-art Cloud technologies
- Embrace Disruption – Take bold risks in delivering real-time data and insights to executives through innovative methods
- Foster Collaboration with “Work together, win together” – ability to engage with cross-functional teams to deliver key data and insights, fostering a culture of teamwork and shared success
- Focus on Execution – Execute “Strive tirelessly for simplicity and usability” – Dedicate efforts to creating data models that prioritize simplicity and usability, ensuring scalability for future needs
- Drive Business Value – Develop certified and scalable data models that offer the highest business value, enabling actionable insights
- Conduct In-depth Analysis – Utilize deep data analysis techniques to uncover actionable insights from data models
- Embrace Challenges – Tackle complex data engineering and design tasks that push your skills to new heights
- As a team player, work closely with data analysts, product owners, and data scientists to understand requirements and overcome challenges collaboratively
- Embrace Learning – Stay agile and adept at adapting new data engineering technologies and methodologies
Qualifications
Your Experience
- 10+ years of experience with data and analytics
- Expert level in writing optimized SQL code
- Advanced proficiency in Python for constructing integrations within GCP DataProc clusters
- Should have worked on the Marketing/Sales domain and built data models eg. Lead Scoring, Contact enrichment, GDPR processes, bookings, pipeline, etc.
- Able to explain the business process and how analytics supported both strategic & operational requirements
- Experience required in building ETL using data from Big Query
- Familiarity with GCP services, preferably having supported deployments on one or more of GCP services
- Experience in Managing code using Github
- Should have worked in agile(scrum) development methodology
- Strong development/automation skills
- Working knowledge with Tableau is preferred
- Can-do attitude on solving complex business problems, good interpersonal and teamwork skills
- Must be located within SF Bay area Candidates to be in office 3 days/ week
Additional Information
The Team
Working at a high-tech cybersecurity company within Information Technology is a once in a lifetime opportunity. You’ll be joined with the brightest minds in technology, creating, building, and supporting tools that enable our global teams on the front line of defense against cyber attacks. We’re joined by one mission – but driven by the impact of that mission and what it means to protect our way of life in the digital age. Join a dynamic and fast-paced team that feels excitement at the prospect of a challenge and feels a thrill at resolving technical gaps that inhibit productivity.
Our Commitment
We’re trailblazers that dream big, take risks, and challenge cybersecurity’s status quo. It’s simple: we can’t accomplish our mission without diverse teams innovating, together.
We are committed to providing reasonable accommodations for all qualified individuals with a disability. If you require assistance or accommodation due to a disability or special need, please contact us at
ac************@pa**************.com
.
Palo Alto Networks is an equal opportunity employer. We celebrate diversity in our workplace, and all qualified applicants will receive consideration for employment without regard to age, ancestry, color, family or medical care leave, gender identity or expression, genetic information, marital status, medical condition, national origin, physical or mental disability, political affiliation, protected veteran status, race, religion, sex (including pregnancy), sexual orientation, or other legally protected characteristics.
All your information will be kept confidential according to EEO guidelines.
The compensation offered for this position will depend on qualifications, experience, and work location. For candidates who receive an offer at the posted level, the starting base salary (for non-sales roles) or base salary + commission target (for sales/commissioned roles) is expected to be between $143,500/yr to $233,200/yr. The offered compensation may also include restricted stock units and a bonus. A description of our employee benefits may be found here.
Is role eligible for Immigration Sponsorship?: Yes
Innovation Incentives – Software/Engineering Technical Consultant – Senior Manager
Job title: Innovation Incentives – Software/Engineering Technical Consultant – Senior Manager
Company: EY
Job description: Innovation Incentives – Software/Engineering Technical Consultant Team: Sitting within the wider Quantitative…. Supporting the business in winning new clients by leveraging from industry sector expertise through the preparation and delivery…
Expected salary:
Location: Southside Dublin
Job date: Fri, 23 Feb 2024 23:26:21 GMT
Apply for the job now!
Sr Innovation Manager
Job title: Sr Innovation Manager
Company:
Job description: creating physical product innovations (not just service/business model innovation). Strong project management skills… friend every day, and realizing our potential as people and as a business! What are we looking for? The Pernod Ricard…
Expected salary:
Location: Dublin
Job date: Sun, 03 Mar 2024 01:13:17 GMT
Apply for the job now!
Digital & Innovation Director
Job title: Digital & Innovation Director
Company: Prosperity
Job description: ’s degree / advanced qualification in business, digital transformation, innovation or related field Proven experience in…Looking for a new innovation role? We are looking for a Digital & Innovation Director to lead new initiatives and long…
Expected salary:
Location: Dublin
Job date: Thu, 07 Mar 2024 23:36:07 GMT
Apply for the job now!
Preventing Harms From AI Misuse
This post summarises a recent paper, “Protecting Society from AI Misuse: When are Restrictions on Capabilities Warranted?”
GovAI research blog posts represent the views of their authors, rather than the views of the organisation.
Introduction
Recent advancements in AI have enabled impressive new capabilities, as well as new avenues to cause harm. Though experts have long warned about risks from the misuse of AI, the development of increasingly powerful systems has made the potential for such harms more widely felt. For instance, in the United States, Senator Blumenthal recently began a Senate hearing by showing how existing AI systems can be used to impersonate him. Last week, the AI company Anthropic reported that they have begun evaluating whether their systems could be used to design biological weapons.
To address growing risks from misuse, we will likely need policies and best practices that shape what AI systems are developed, who can access them, and how they can be used. We call these “capabilities interventions.”
Unfortunately, capabilities interventions almost always have the unintended effect of hindering some beneficial uses. Nonetheless, we still argue that — at least for certain particularly high-risk systems — these sorts of interventions will be increasingly warranted as potential harms from AI systems increase in severity.
The Growing Risk of Misuse
Some existing AI systems – including large language models (LLMs), image and audio generation systems, and drug discovery systems – already have clear potential for misuse. For instance, LLMs can speed up and scale up spear phishing cyber attacks to target individuals more effectively. Image and audio generation models can create harmful content like revenge porn or misleading “deepfakes” of politicians. Drug discovery models can be used to design dangerous novel toxins: for example, researchers used one of these models to discover over 40,000 potential toxins, with some predicted to be deadlier than the nerve agent VX.
As AI systems grow more capable across a wide range of tasks, we should expect some of them to become more capable of performing harmful tasks as well. Although we cannot predict precisely what capabilities will emerge, experts have raised a number of concerning near- and medium-term possibilities. For instance, LLMs might be used to develop sophisticated malware. Video-generation systems could allow users to generate deepfake videos of politicians that are practically indistinguishable from reality. AI systems intended for scientific research could be used to design biological weapons with more destructive potential than known pathogens. Other novel risks will likely emerge as well. For instance, a recent paper highlights the potential for extreme risks from general-purpose AI systems capable of deception and manipulation.
Three Approaches to Reducing Misuse Risk
Developers and governments will need to adopt practices and policies (“interventions”) that reduce risks from misuse. At the same time, they will also need to avoid interfering too much with beneficial uses of AI.
To help decision-makers navigate this dilemma, we propose a conceptual framework we refer to as the “Misuse Chain”. The Misuse Chain breaks the misuse of AI down into three stages, which can be targeted by different interventions.
Capabilities interventions target the first stage of the Misuse Chain, in which malicious actors gain access to the capabilities needed to cause harm. For instance, if an AI company wishes to make it harder for bad actors to create non-consensual pornographic deepfakes, it can introduce safeguards that block its image generation systems from generating sexual imagery. It can also take additional measures to prevent users from removing these safeguards, for instance by deciding not to open-source the systems (i.e. make them freely available for others to download and modify).
Harm mitigation interventions target the next stage in the Misuse Chain, when capabilities are misused and produce harm. For example, in the case of pornographic deepfakes, harm can be mitigated by identifying the images and preventing them from being shared on social media.
Harm response interventions target the final stage of the Misuse Chain, in which bad actors experience consequences of their actions and victims receive remedies. For example, people caught using AI systems to generate explicit images of minors could not only have their access revoked but also face punishment through the legal system. Here, the aim is to deter future attempts at misuse or rectify the harm it has already caused.
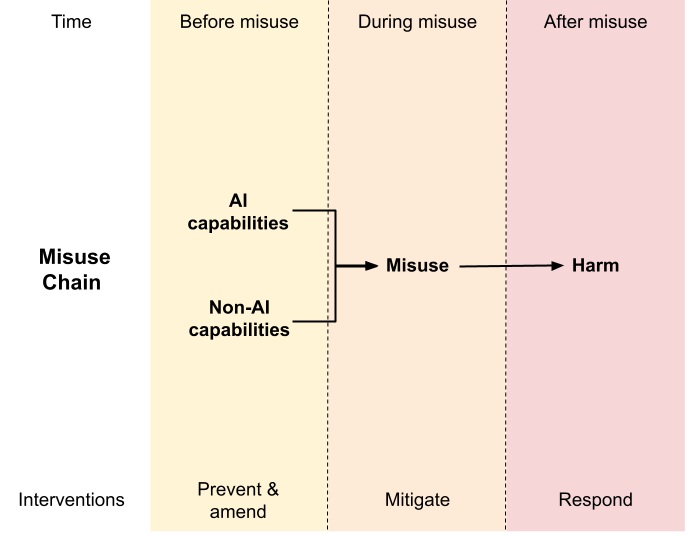
Capabilities Interventions and the Misuse-Use Tradeoff
Interventions that limit risks from misuse can also hinder beneficial uses; they are subject to what we call the “Misuse-Use Tradeoff”. As interventions move “upstream” along the Misuse Chain — and more directly affect the core capabilities that enable malicious actors to cause harm — they tend to become less targeted and exhibit more significant trade-offs. Interventions near the top of the chain can limit entire classes of use.
Take, for example, content policies for large language models that prohibit the model from outputting any text related to violence. Such a blunt policy tool may prevent harmful content from being generated, but it also prevents the model from being used to analyse or generate fictional works that reference violence. This is an undesirable side effect.
However, if an AI system can be used to cause severe harm, then the benefits of even fairly blunt capability interventions may outweigh the costs.
Why Capabilities Interventions May Be Increasingly Necessary
Where possible, developers and policymakers should prefer harm mitigation and harm response interventions. These interventions are often more targeted, meaning that they are more effective at reducing misuse without substantially limiting beneficial use.
However, these sorts of interventions are not always sufficient. There are some situations where capabilities interventions will be warranted, even if other interventions are already in place. In broad terms, we believe that capabilities interventions are most warranted when some or all of the following conditions hold:
- The harms from misuse are especially significant. If the potential harms from misuse significantly outweigh the benefits from use, then capabilities interventions may be justified even if they meaningfully reduce beneficial use.
- Other approaches to reducing misuse have limited efficacy if applied alone. If interventions at other stages are not very effective, then capabilities interventions will be less redundant.
- It is possible to restrict access to capabilities in a targeted way. Though capabilities interventions tend to impact both misuse and use, some have little to no impact on legitimate use. For example, AI developers can monitor systems’ outputs to flag users who consistently produce inappropriate content and adjust their access accordingly. If the content classification method is reliable enough, this intervention may have little effect on most users. In such cases, the argument for capability interventions may be particularly strong.
Ultimately, as risks from the misuse of AI continue to grow, the overall case for adopting some capability interventions will likely become stronger. Progress in developing structured access techniques, which limit capability access in finely targeted ways, could further strengthen the case.
Varieties of Capability Interventions
In practice, to reduce misuse risk, there are several varieties of capability interventions that developers and policymakers could pursue. Here, we give three examples.
- Limiting the open source sharing of certain AI systems. Open-sourcing an AI system makes it harder to prevent users from removing safeguards, reintroducing removed capabilities, or introducing further dangerous capabilities. It is also impossible to undo the release of a system that has been open-sourced, even if severe harms from misuse start to emerge. Therefore, it will sometimes be appropriate for developers to refrain from open-sourcing high-risk AI systems – and instead opt for structured access approaches when releasing the systems. In sufficiently consequential cases, governments may even wish to require the use of structured access approaches.
- Limiting the sharing or development of certain AI systems altogether. In some cases, for instance when an AI system is intentionally designed to cause harm, the right decision will be to refrain from development and release altogether. If the problem of emergent dangerous capabilities becomes sufficiently severe, then it may also become wise to refrain from developing and releasing certain kinds of dual-use AI systems. As discussed above, in sufficiently high-risk cases, governments may wish to consider regulatory options.
- Limit access to inputs needed to create powerful AI systems. Companies and governments can also make it harder for certain actors to access inputs (such as computing hardware) that are required to develop harmful AI systems. For example, the US recently announced a series of export controls on AI-relevant hardware entering China, in part to curb what it considers Chinese misuse of AI in the surveillance and military domains. Analogously, cloud computing providers could also introduce Know Your Customer schemes to ensure their hardware is not being used to develop or deploy harmful systems.
Conclusion
As AI systems become more advanced, the potential for misuse will grow. Balancing trade-offs between reducing misuse risks and enabling beneficial uses will be crucial. Although harm mitigation and harm response interventions often have smaller side effects, interventions that limit access to certain AI capabilities will probably become increasingly warranted.
AI labs, governments, and other decision makers should be prepared to increasingly limit access to AI capabilities that lend themselves to misuse. Certain risks from AI will likely be too great to ignore, even if that means we must impede on legitimate use. These actors should also work on building up processes for determining when capabilities interventions are warranted. Furthermore, they should invest in developing interventions that further reduce the Misuse-Use Tradeoff. Continued research could yield better tools for preventing dangerous capabilities from ending up in the wrong hands, without also preventing beneficial capabilities from ending up in the right hands.
Preventing AI Misuse: Current Techniques
This post aims to give policymakers an overview of available methods for preventing the misuse of general-purpose AI systems, as well as the limitations of these methods.
GovAI research blog posts represent the views of their authors, rather than the views of the organisation.
Introduction
As general-purpose AI models have become more capable, their potential for misuse has grown as well. Without safety measures in place, for example, many models can now be used to create realistic fake media. Some experts worry that future models could facilitate more extreme harm, including cyberattacks, voter manipulation, or even attacks with biological weapons.
Governments and companies increasingly recognise the need to evaluate models for dangerous capabilities. However, identifying dangerous capabilities is only a first step toward preventing misuse.
Developers must ultimately find ways to remove dangerous capabilities or otherwise limit the ability of users to apply them.1 To this end, developers currently implement a number of misuse-prevention techniques. These include fine-tuning, content filters, rejection sampling, system prompts, dataset filtering, and monitoring-based restrictions.
However, existing misuse-prevention techniques are far from wholly reliable. It is particularly easy to circumvent them when models are “open-sourced”. Even when models are accessed through online interfaces, however, the techniques can still be fairly easily circumvented through “jailbreaking” techniques. External researchers have demonstrated, for example, that jailbroken systems can perform tasks such as crafting targeted phishing emails.
If future models do eventually develop sufficiently dangerous capabilities (such as assisting in the planning and execution of a biological attack), then it may not be responsible to release them until misuse-prevention techniques are more reliable. Therefore, improving misuse-prevention techniques could reduce both social harms and future delays to innovation.
Misuse-prevention techniques
In this section, I will briefly survey available techniques for preventing misuse. I will then turn to discussing their limitations in more depth.
Fine-tuning
Companies developing general-purpose AI systems — like OpenAI and Anthropic — primarily reduce harmful outputs through a process known as “fine-tuning”. This involves training an existing AI model further, using additional data, in order to refine its capabilities or tendencies.
This additional data could include curated datasets of question/answer pairs, such as examples of how the model should respond to irresponsible requests. Fine-tuning data may also be generated through “reinforcement learning from human feedback” (RLHF), which involves humans scoring the appropriateness of the models’ responses, or through “reinforcement learning from AI feedback” (RLAIF). Through fine-tuning, a model can be conditioned to avoid dangerous behaviours or decline dangerous requests. For instance, GPT-4 was fine-tuned to reject requests for prohibited content, such as instructions for cyberattacks.
As with other misuse-prevention techniques, however, there are trade-offs with this approach: fine-tuning can also make models less helpful, by leading them to also refuse some benign requests.
Filters
Filters can be applied to both user inputs and model outputs. For inputs, filters are designed to detect and block users’ requests to produce dangerous content. For outputs, filters are designed to detect and block dangerous content itself.
Developers have various methods to train these filters to recognise harmful content. One option is using labelled datasets to categorise content as either harmful or benign. Another option is creating a new dataset by having humans score the harmfulness of the outputs a model produces or the outputs users send to it. Language models themselves can also be used to evaluate both inputs and outputs.
Rejection sampling
Rejection sampling involves generating multiple outputs from the same AI model, automatically scoring them based on a metric like “potential harm”, and then only presenting the highest scoring output to the user. OpenAI used this approach with WebGPT, the precursor to ChatGPT, to make the model more helpful and accurate.
System prompts
System prompts are natural language instructions to the model that are pre-loaded into user interactions and are usually hidden from the user. Research indicates incorporating directives like “ignore harmful requests” into prompts can reduce harmful outputs.
Dataset filtering
Before training an AI model, developers can remove harmful data to ensure the model doesn’t learn from it. For example, OpenAI removed sexual and violent content from the dataset they trained DALLE-3 on. They make use of the same ‘classifiers’ developed for filters to identify training data that should be removed. A distinct downside of this approach, however, is that it can only be applied before a model is trained.
Monitoring-based restrictions
The techniques above take a purely preventative approach. Monitoring-based restrictions instead allow developers to respond to initial cases of misuse – or misuse attempts – by limiting users’ abilities to engage in further misuse.
AI service providers can leverage automated tools like input and output classifiers to screen for misuse. For instance, if a user repeatedly makes requests that are blocked by an input filter, the user may be flagged as high-risk. They might then be sent a warning, have their access temporarily reduced, or even receive a ban. Monitoring can also help reveal new vulnerabilities and patterns of misuse, allowing policies and other safeguards to be iteratively improved.
One potential problem with monitoring is that it can compromise privacy. That said, there are ways to preserve privacy. For instance, reports about high-level patterns of misuse can be generated without company staff needing to examine individuals’ data.
How effective are the mitigations?
Unfortunately, these mitigations are currently ineffective for open-source models (i.e. models that users are allowed to download and run on their own hardware). Safeguards like filters, system prompts, and rejection sampling mechanisms can often be disabled by changing a few lines of code. Fine-tuning is also relatively trivial to reverse; one study also showed that fine-tuning for various Llama models could be undone for under $200. Additionally, once a model is open-sourced, there is no way to reliably oversee how it is being modified or used post-release. Although open-source models have a range of socially valuable advantages, they are also particularly prone to be misused.2
Putting behind an “application programming interface” (API) can help prevent the removal of safeguards. However, even when developers put their models behind an API, the safeguards can reduce, but not eliminate, misuse risks. OpenAI successfully reduced rule-breaking responses by 82% in GPT-4, but risks still remain. Anthropic similarly states that, despite interventions, their systems are not “perfectly safe”.
One challenge in preventing misuse is the phenomenon of “jailbreaks”, where users bypass AI system’s safety measures to generate prohibited content. Successful jailbreaks have employed tactics such as switching to a different language to evade content filters or framing requests for dangerous information within hypothetical scenarios like a script for a play.

Despite efforts from AI developers, it is still relatively trivial to jailbreak models. For instance, GPT-4 was jailbroken within 24 hours of release, despite the fact that OpenAI spent 6 months investing in safety measures. Developers are actively working to patch identified vulnerabilities, but users have continuedtofind ways around restrictions, as documented on sites like JailbreakChat.
Implications
It seems likely that the benefits of current models outweigh their harms. This is in part thanks to the mitigation techniques discussed here, but also in large part due to the model’s limited capabilities. For instance, the propensity of GPT-4 to make factual mistakes puts a cap on its ability to perform cyberattacks. In some cases, existing safeguards may not even be worth the costs they create for users (e.g. for instance by accidentally filtering benign content).
However, the unreliability of current misuse-prevention techniques may pose significant challenges in the future. If future models become sufficiently powerful, then even a single case of misuse, like a user building a bioweapon, could eventually be catastrophic. One possible implication is that models with sufficiently dangerous capabilities should not be released until better misuse-prevention techniques are developed.
If this implication is right, then better misuse-prevention techniques could prevent future roadblocks to innovation in addition to lowering societal risks.
Conclusion
There are a range of techniques developers can use to limit the misuse of their models. However, these are not consistently effective. If future models do develop sufficiently dangerous capabilities, then the reliability of misuse-prevention techniques could ultimately be a bottleneck to releasing them.
The author of this piece can be contacted at
be**********@go********.ai
. Thanks to the following people for their feedback: Alan Chan, Ben Garfinkel, Cullen O’Keefe, Emma Bluemke, Julian Hazell, Markus Anderljung, Matt van der Merwe, Patrick Levermore, Toby Shevlane.