Job title: Business Insurance Specialist
Company: Radius Payment Solutions
Job description: of technology innovation and we invite you along on this journey. The role … We are currently looking for a Business Insurance…, forward-thinking global business who build transformative solutions for our customers to deliver best-in-class sustainable mobility…
Expected salary: €27500 per year
Location: Dundalk, Co Louth
Job date: Wed, 15 May 2024 05:14:50 GMT
Apply for the job now!
Summer Fellowship 2022 Wrap Up – What Did Our Fellows Work On?
As our 2022 Summer Fellowship comes to a close, we’re proud to highlight what our Summer Fellows have been up to!
Summer and Winter Fellowships provide an opportunity for early-career individuals to spend three months working on an AI governance research project, learning about the field, and making connections with other researchers and practitioners.
Summer Fellows come from a variety of disciplines and a range of prior experience – some fellows ventured into entirely new intellectual territory for their projects, and some fellows used the time to extend their previous work.
We gratefully thank all of the supervisors for their mentorship and guidance this summer, and for dedicating time to training the next generation of researchers.
If you’re interested in applying for future fellowships, check out our Opportunities page. You can register your expression of interest here.
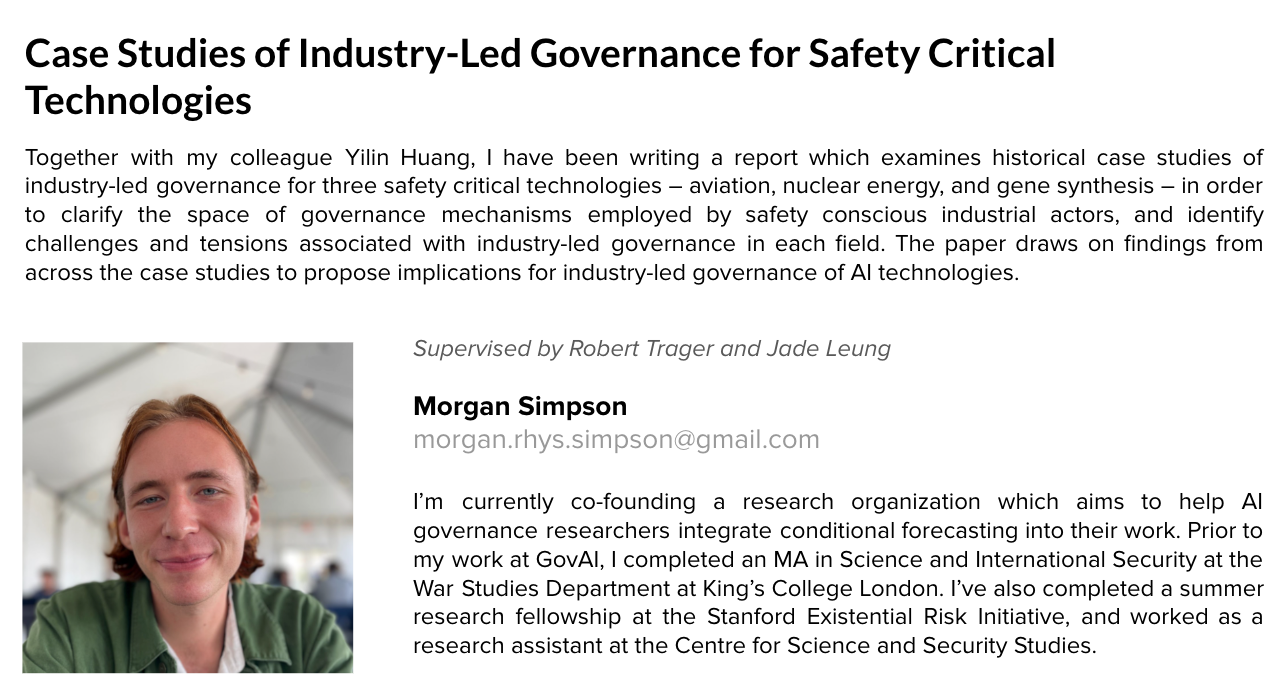
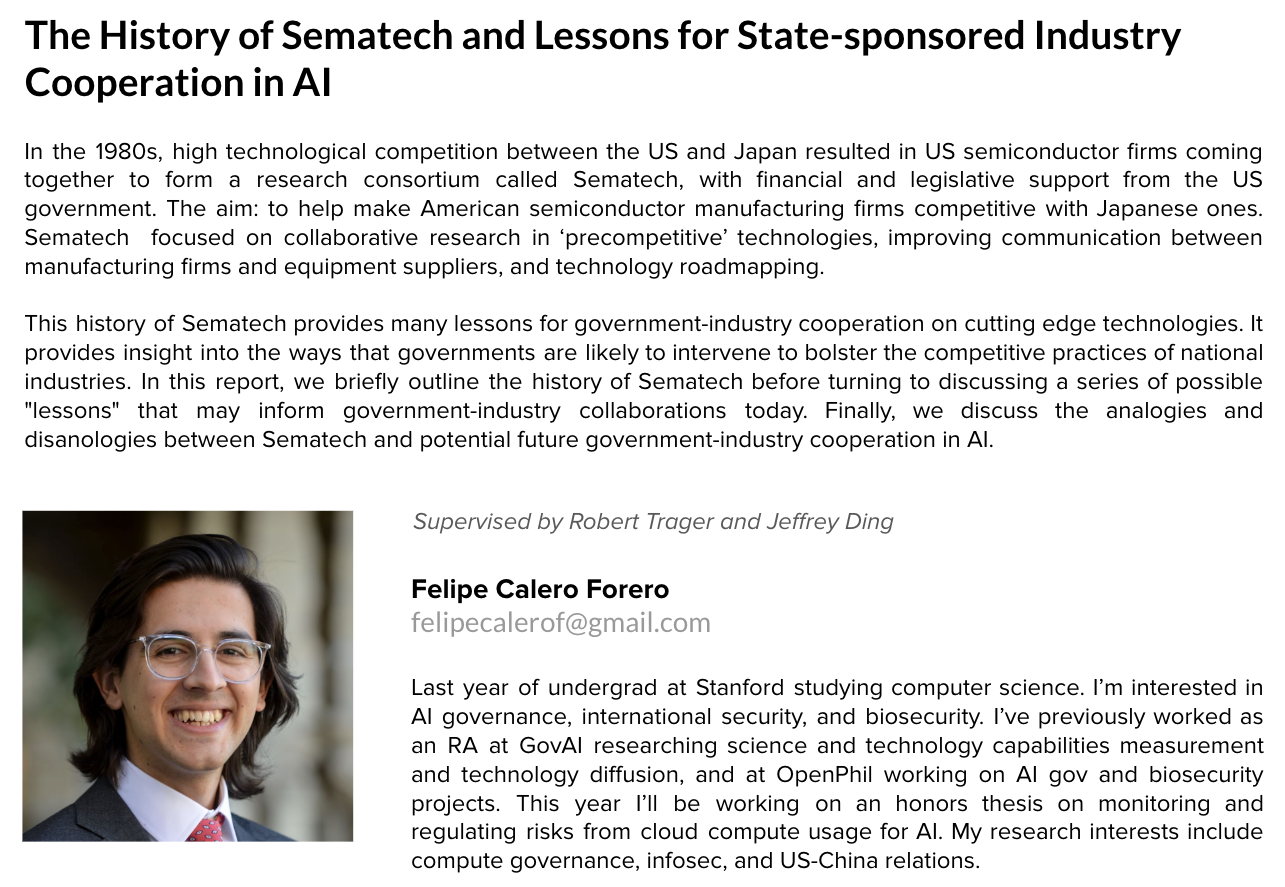
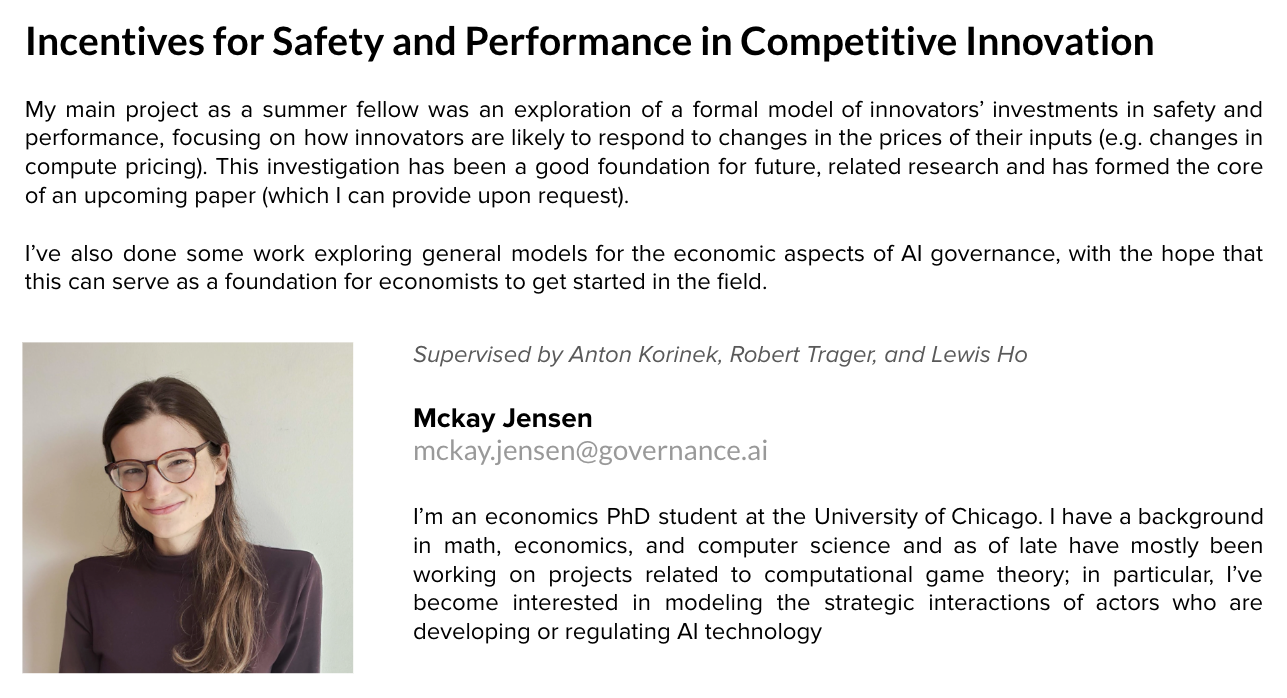
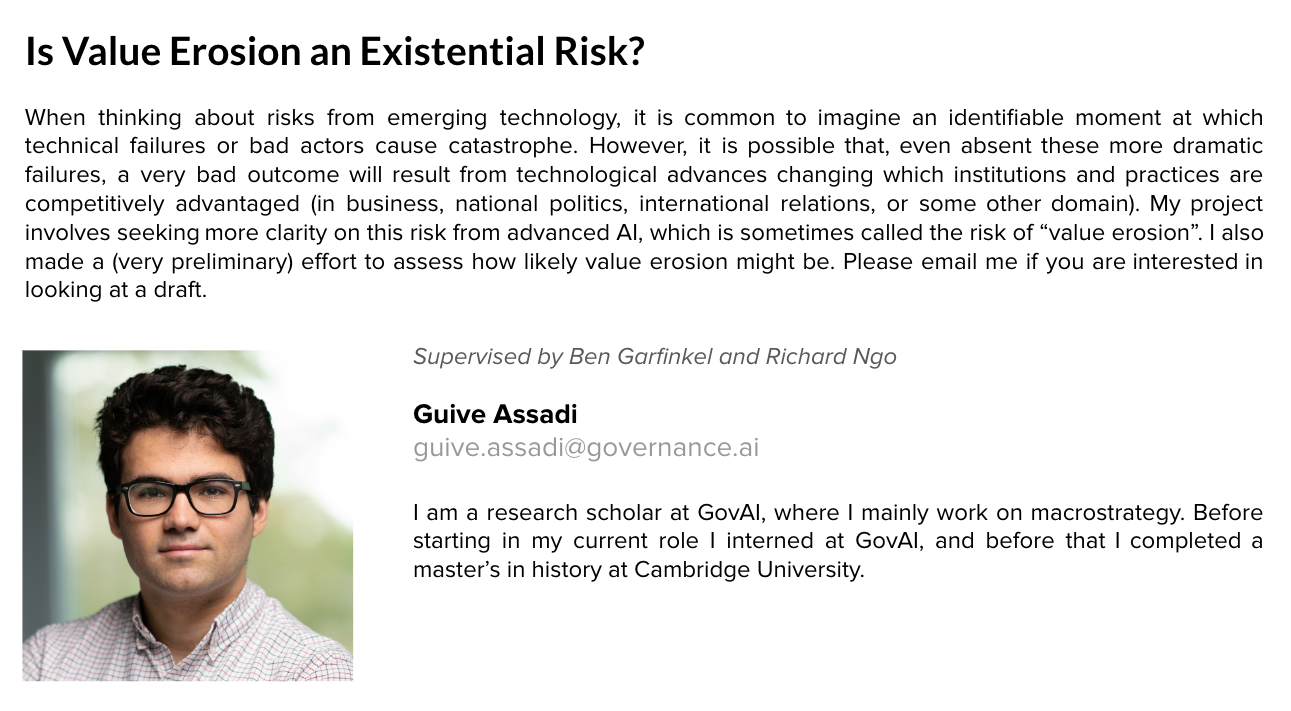
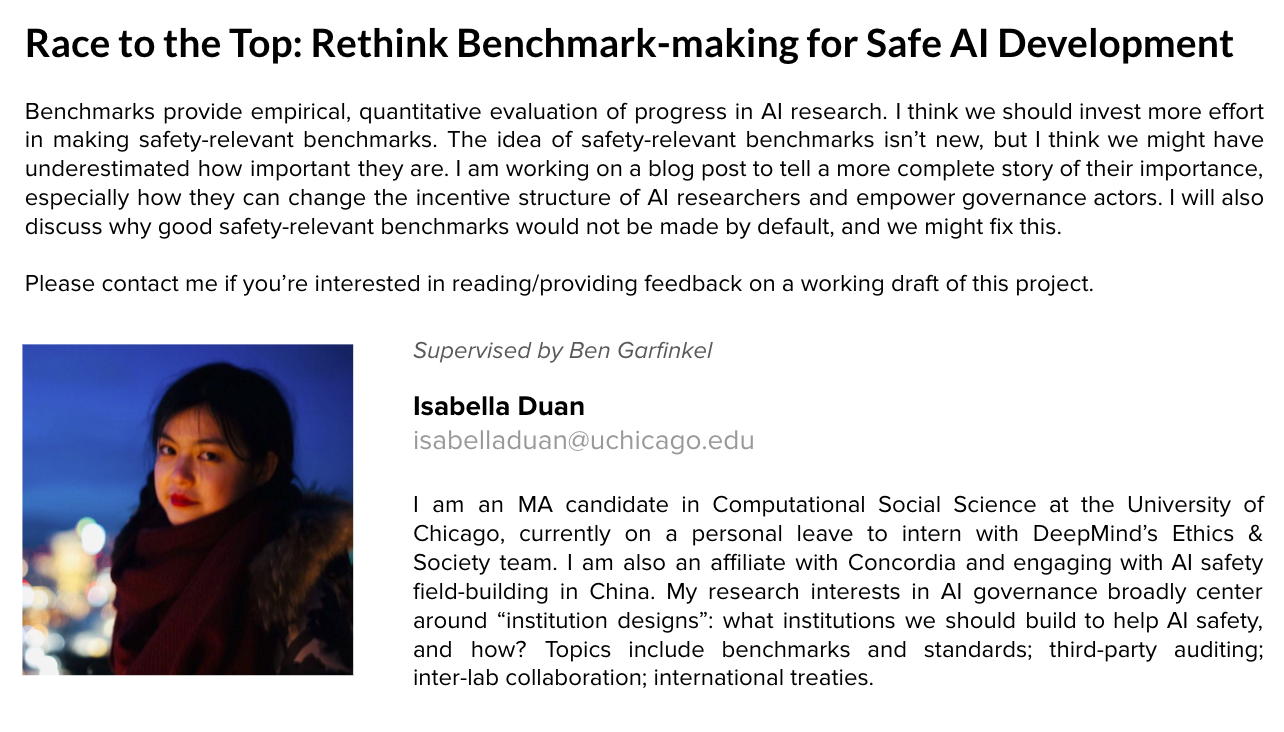
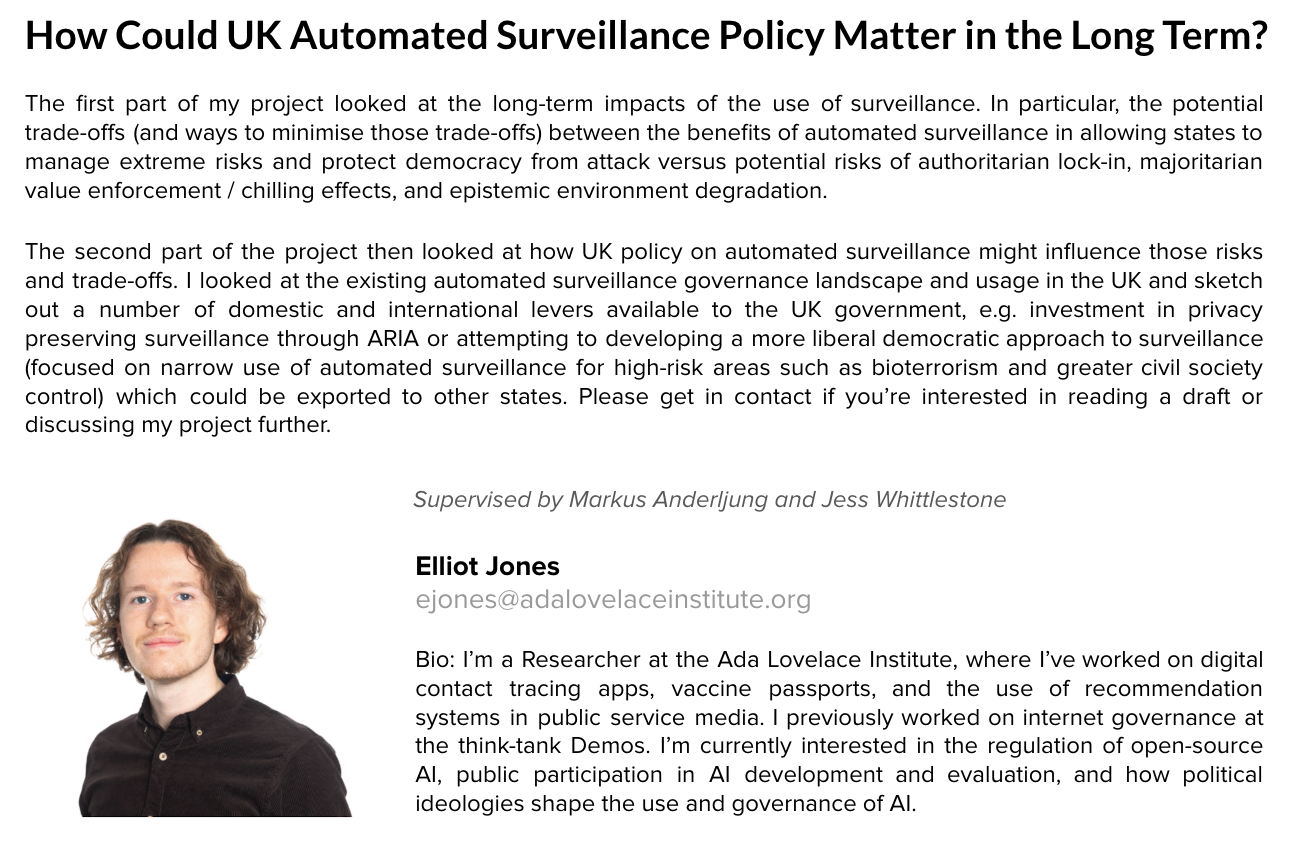
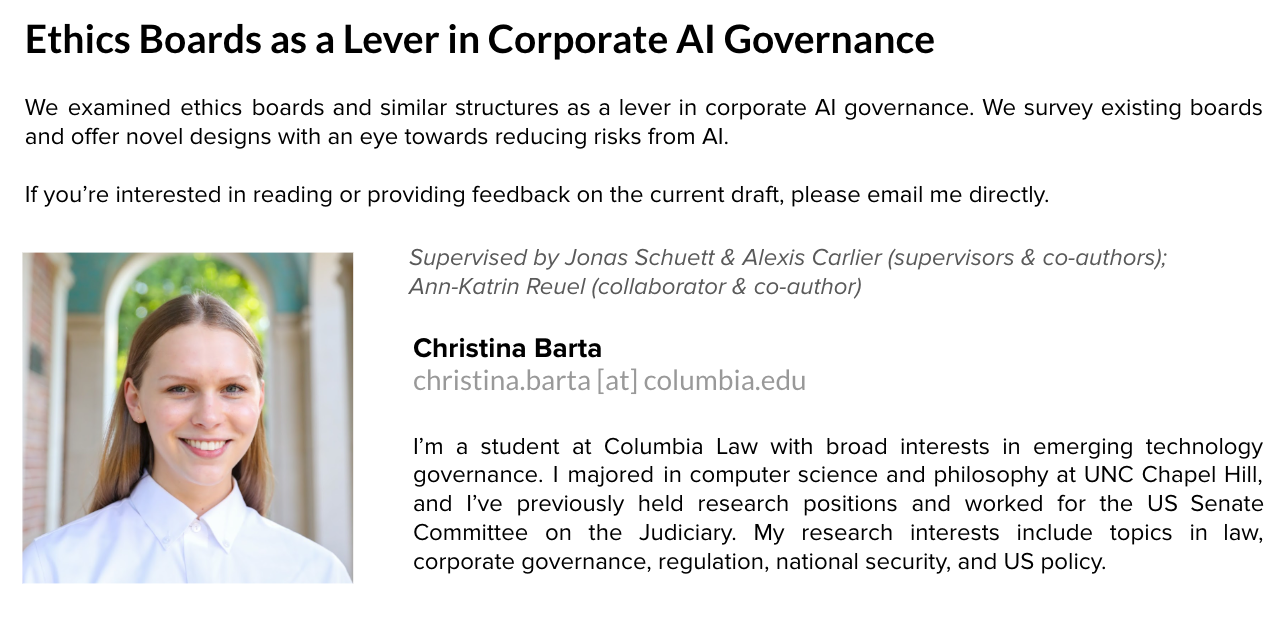

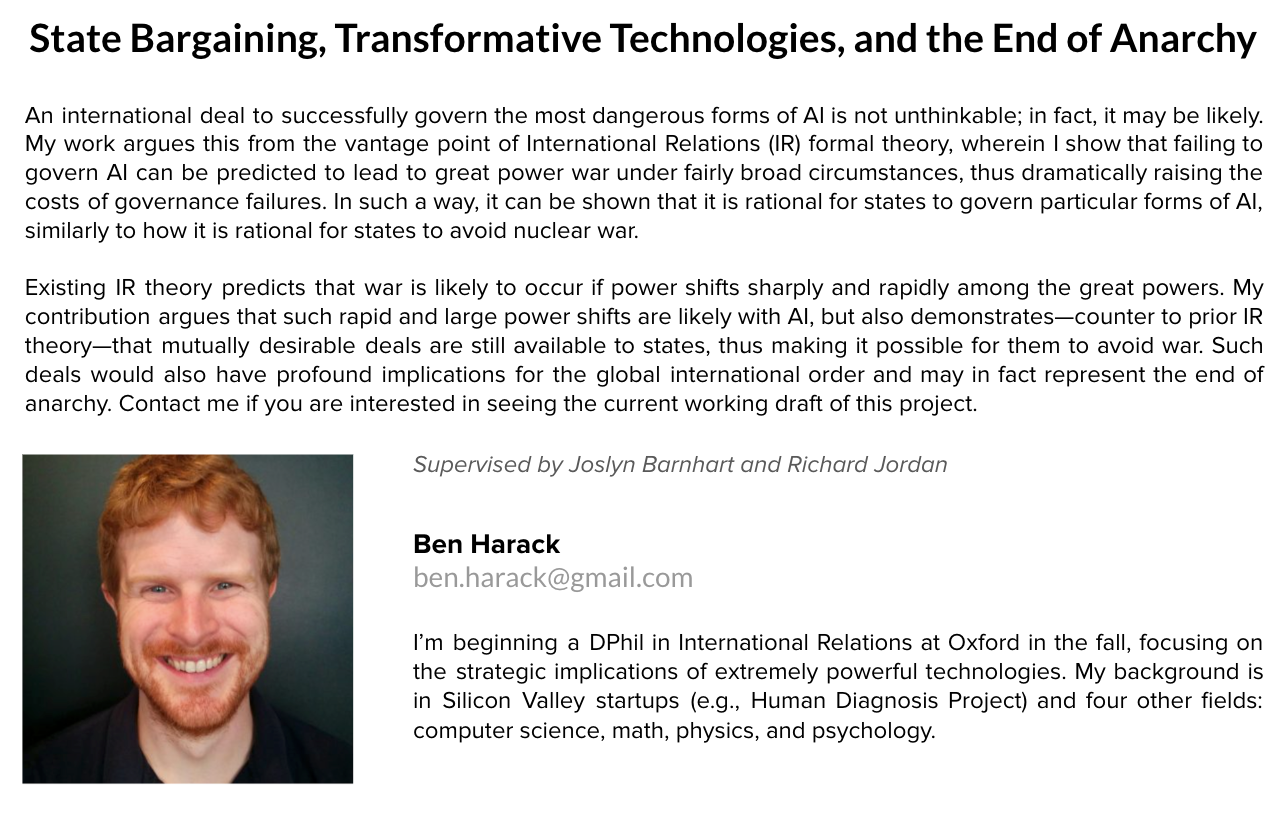
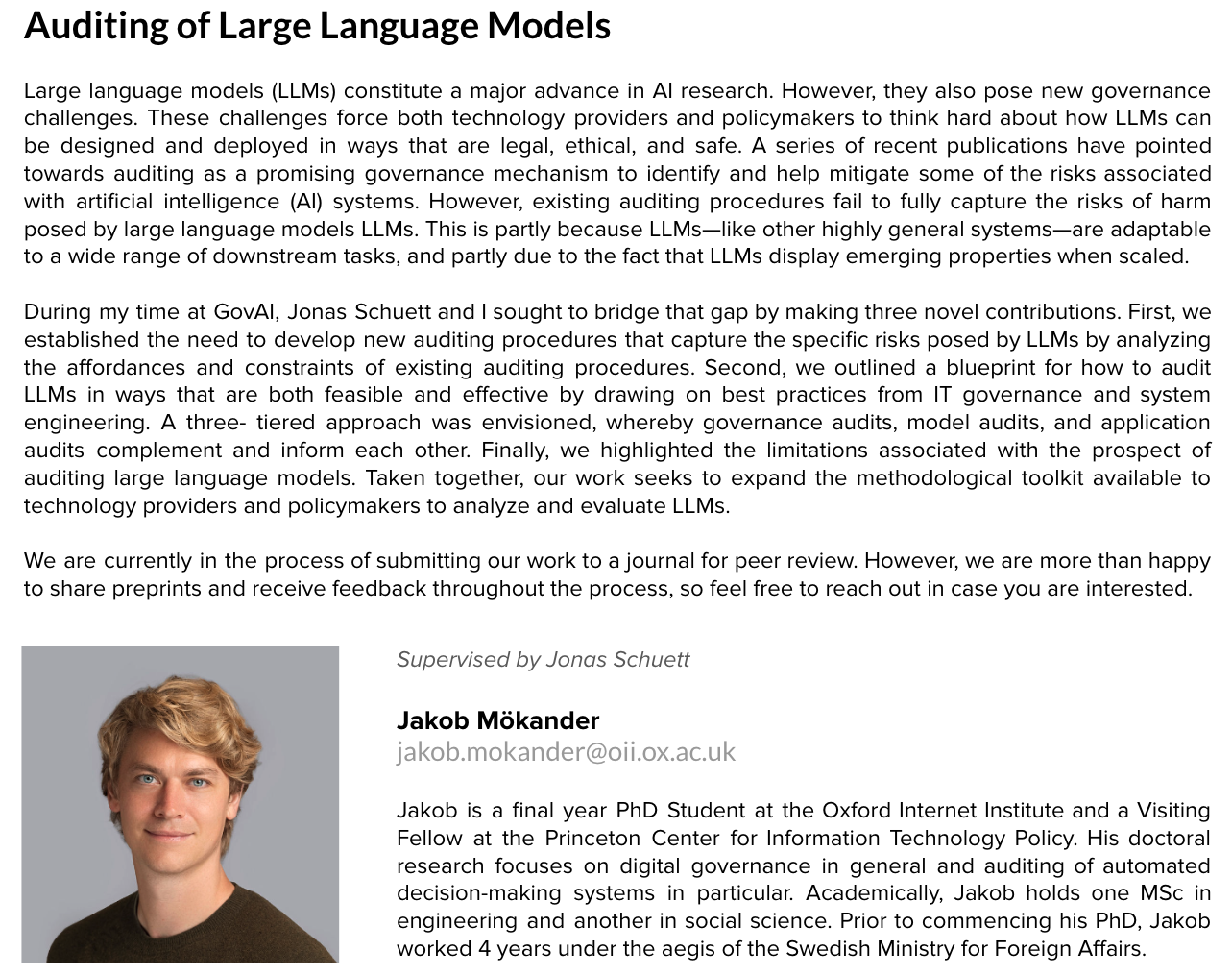
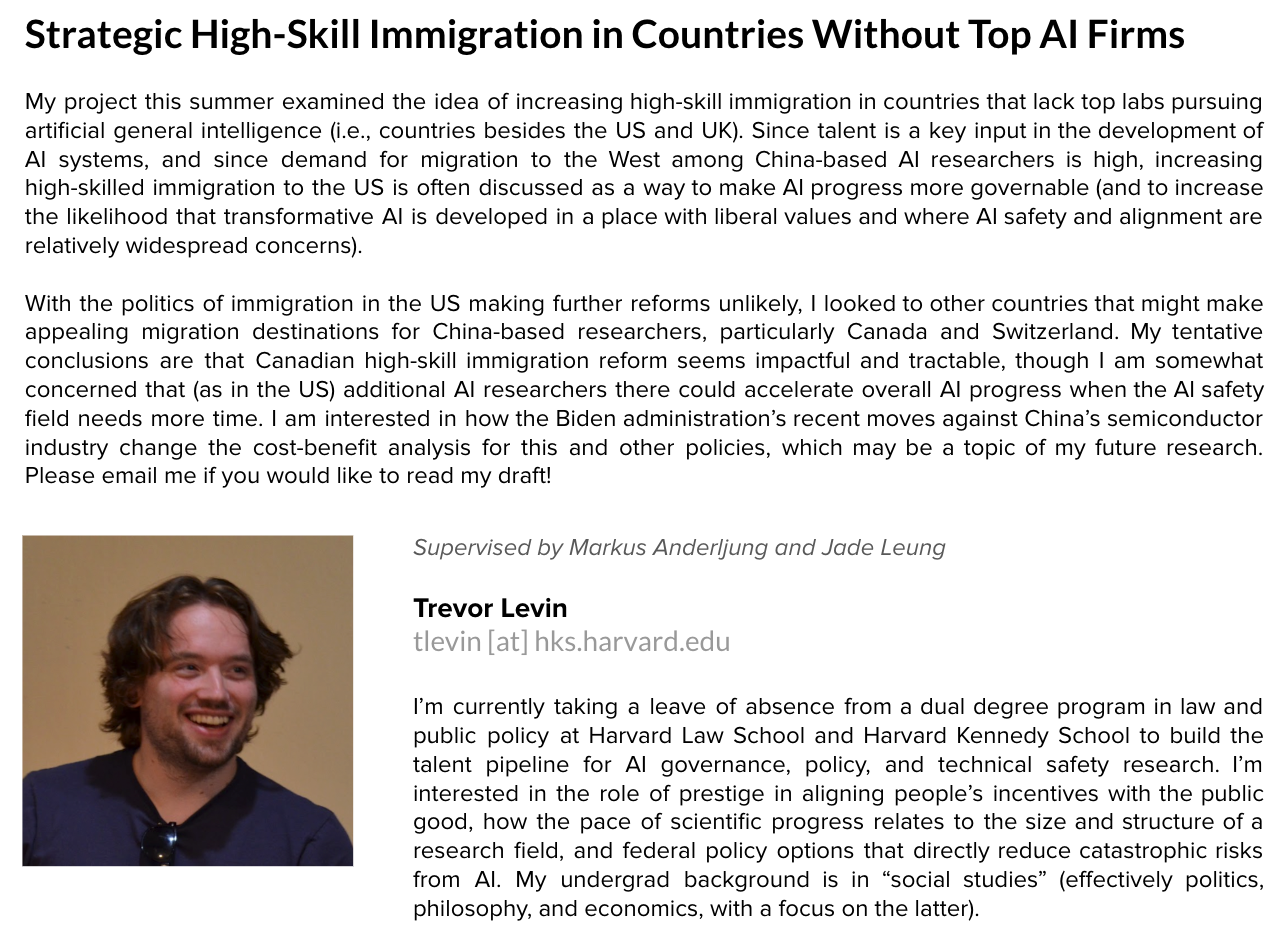
Graduate Sales & Business Management Trainee
Job title: Graduate Sales & Business Management Trainee
Company: Bridgewater UK
Job description: Are you a recent graduate with a passion for business and the drive to succeed? The UK and Ireland’s largest supplier… of electrical equipment to business and trade customers is offering an exciting opportunity to join their comprehensive graduate…
Expected salary:
Location: Ireland
Job date: Wed, 15 May 2024 05:22:14 GMT
Apply for the job now!
Open Problems in Cooperative AI
Read the paper “Open Problems in Cooperation” here. You can also read about the work of the Cooperative AI Foundation, whose work was inspired by the paper, on their website. The following extended abstract summarizes the paper’s key message:
Problems of cooperation—in which agents have opportunities to improve their joint welfare but are not easily able to do so—are ubiquitous and important. They can be found at all scales ranging from our daily routines—such as driving on highways, scheduling meetings, and working collaboratively—to our global challenges—such as peace, commerce, and pandemic preparedness. Human civilization and the success of the human species depends on our ability to cooperate.
Advances in artificial intelligence pose increasing opportunity for AI research to promote human cooperation. AI research enables new tools for facilitating cooperation, such as language translation, human-computer interfaces, social and political platforms, reputation systems, algorithms for group decision-making, and other deployed social mechanisms; it will be valuable to have explicit attention to what tools are needed, and what pitfalls should be avoided, to best promote cooperation. AI agents will play an increasingly important role in our lives, such as in self-driving vehicles, customer assistants, and personal assistants; it is important to equip AI agents with the requisite competencies to cooperate with others (humans and machines). Beyond the creation of machine tools and agents, the rapid growth ofAI research presents other opportunities for advancing cooperation, such as from research insights into social choice theory or the modeling of social systems.
The field of artificial intelligence has an opportunity to increase its attention to this class of problems, which we refer to collectively as problems in Cooperative AI. The goal would be to study problems of cooperation through the lens of artificial intelligence and to innovate in artificial intelligence to help solve these problems. Whereas much AI research to date has focused on improving the individual intelligence of agents and algorithms, the time is right to also focus on improving social intelligence: the ability of groups to effectively cooperate to solve the problems they face.
AI research relevant to cooperation has been taking place in many different areas, including in multi-agent systems, game theory and social choice, human-machine interaction and alignment, natural language processing, and the construction of social tools and platforms. Our recommendation is not merely to construct an umbrella term for these areas, but rather to encourage focused research conversations, spanning these areas, focused on cooperation. We see opportunity to construct more unified theory and vocabulary related to problems of cooperation. Having done so, we think AI research will be in a better position to learn from and contribute to the broader research program on cooperation spanning the natural sciences, social sciences, and behavioural sciences.
Our overview comes from the perspective of authors who are especially impressed by and immersed in the achievements of deep learning(1) and reinforcement learning (2). From that perspective, it will be important to develop training environments, tasks, and domains that can provide suitable feedback for learning and in which cooperative capabilities are crucial to success, non-trivial, learnable, and measurable. Much research in multi-agent systems and human-machine interaction will focus on cooperation problems in contexts of pure common interest. This will need to be complemented by research in mixed-motives contexts, where problems of trust, deception, and commitment arise. Machine agents will often act on behalf of particular humans and will impact other humans; as a consequence, this research will need to consider how machines can adequately understand human preferences, and how best to integrate human norms and ethics into cooperative arrangements. Researchers building social tools and platforms will have other perspectives on how best to make progress on problems of cooperation, including being especially informed by real-world complexities. Areas such as trusted hardware design and cryptography may be relevant for addressing commitment problems and cryptography. Other aspects of the problem will benefit from expertise from other sciences, such as political science, law, economics, sociology, psychology, and neuroscience. We anticipate much value in explicitly connecting AI research to the broader scientific enterprise studying the problem of cooperation and to the broader effort to solve societal cooperation problems.
We recommend that “Cooperative AI” be given a technically precise, problem-defined scope; otherwise, there is a risk that it acquires an amorphous cloud of meaning, incorporating adjacent (clusters of) concepts such as aligned AI, trustworthy AI, and beneficial AI. Cooperative AI, as scoped here, refers to AI research trying to help individuals, humans and machines, to find ways to improve their joint welfare.For any given situation and set of agents, this problem is relatively well defined and unambiguous. The Scope section elaborates on the relationship to adjacent areas. Conversations on Cooperative AI can be organized in part in terms of the dimensions of cooperative opportunities. These include the strategic context, the extent of common versus conflicting interest, the kinds of entities who are cooperating, and whether the researchers are focusing on the cooperative competence of individuals or taking the perspective of a social planner. Conversations can also be focused on key capabilities necessary for cooperation, including:
- Understanding of other agents, their beliefs, incentives, and capabilities.
- Communication between agents, including building a shared language and overcoming mistrustand deception.
- Constructing cooperative commitments, so as to overcome incentives to renege on a cooperative arrangement.
- Institutions, which can provide social structure to promote cooperation, be they decentralizedand informal, such as norms, or centralized and formal, such as legal systems.
Just as any area of research can have downsides, so is it prudent to investigate the potential downsides of research on Cooperative AI. Cooperative competence can be used to exclude others, some cooperative capabilities are closely related to coercive capabilities, and learning cooperative competence can be hard to disentangle from coercion and competition. An important aspect of this research, then, will be investigating potential downsides and studying how best to anticipate and mitigate them. The paper is structured as follows. We offer more motivation in the section “Why Cooperative AI?” We then discuss several important dimensions of “Cooperative Opportunities.” The bulk of our discussion is contained in the “Cooperative Capabilities” section, which we organize in terms of Understanding, Communication, Commitment, and Institutions. We then reflect on “The Potential Downsides of Cooperative AI” and how to mitigate them. Finally, we conclude.
To read the full “Open Problems in Cooperative AI” report PDF, click here.
HR Business Partner
Job title: HR Business Partner
Company: Sanderson Recruitment
Job description: HR | Ireland | Contract | € 65000 – € 70000 HR Business Partner Role Summary Sanderson is recruiting… for an experienced HR Business Partner for a fixed term contract for 12 Months responsible for supporting colleagues and senior…
Expected salary: €65000 – 70000 per year
Location: Ireland
Job date: Thu, 16 May 2024 07:49:51 GMT
Apply for the job now!
Tom Davidson on What a Compute-centric Framework Says About Takeoff Speeds | GovAI Blog
In March 2023, we hosted a presentation by Tom Davidson (Open Philanthropy) about his draft paper “What a Compute-Centric Framework Says About Takeoff Speeds”. GovAI’s Acting Director Ben Garfinkel..
Source link
Digital Solution Specialist – Business Applications – SMC – Dutch speaker
Job title: Digital Solution Specialist – Business Applications – SMC – Dutch speaker
Company: Microsoft
Job description: companies do business and how to accelerate innovation and empower the success of our customers. This opportunity will allow… to be Microsoft’s next $100 billion-dollar business – this is where you come in. As part of local subsidiaries or Digital Sales…
Expected salary:
Location: Dublin
Job date: Thu, 16 May 2024 07:51:28 GMT
Apply for the job now!
Compute Funds and Pre-trained Models
This post, authored by Markus Anderljung, Lennart Heim, and Toby Shevlane, argues that a newly proposed US government institution has an opportunity to support “structured access” to large AI models. GovAI research blog posts represent the views of their authors, rather than the views of the organization.
Compute funds and pre-trained models
One of the key trends in AI research over the last decade is its growing need for computational resources. Since 2012, the compute required to train state-of-the-art (SOTA) AI models has been doubling roughly every six months. Private AI labs are producing an increasing share of these high-compute SOTA AI models(1), leading many to worry about a growing compute divide between academia and the private sector. Partly in response to these concerns, there have been calls for the creation of a National AI Research Resource (NAIRR). The NAIRR would help provide academic researchers with access to compute, by either operating its own compute clusters or distributing credits that can be used to buy compute from other providers. It would also further support academic researchers by granting them access to data, including certain government-held datasets. Congress has now tasked the National Science Foundation with setting up a National AI Research Resource Task Force, which is due to deliver an interim report on the potential design of the NAIRR in May 2022.
We argue that for the NAIRR to meet its goal of supporting non-commercial AI research, its design must take into account what we predict will be another closely related trend in AI R&D: an increasing reliance on large pre-trained models, accessed through application programming interfaces (APIs). Large pre-trained models are AI models that require vast amounts of compute to create and that can often be adapted for a wide array of applications. The most widely applicable of these pre-trained models have recently been called foundation models, because they can serve as a “foundation” for the development of many other models. Due to commercial considerations and concerns about misuse, we predict that private actors will become increasingly hesitant to allow others to download copies of these models. We instead expect these models to be accessible primarily through APIs, which allow people to use or study models that are hosted by other actors. While academic researchers need access to compute and large datasets, we argue that they will also increasingly require API access to large pre-trained models. (Others have made similar claims.) The NAIRR could facilitate such access by setting up infrastructure for hosting and accessing large pre-trained models and inviting developers of large pre-trained models (across academia, industry, and government) to make their models available through the system. At the same time, they could allow academics to use NAIRR compute resources or credits to work with these models.
The NAIRR has an opportunity, here, to ensure that academic researchers will be able to learn from and build upon some of the world’s most advanced AI models. Importantly, by introducing an API, the NAIRR could provide structured access to the pre-trained models so as to reduce any risks they might pose, while still ensuring easy access for research use. API access can allow outside researchers to understand and audit these models, for instance identifying security vulnerabilities or biases, without also making it easy for others to repurpose and misuse them.
Concretely, we recommend that the NAIRR:
- provides infrastructure that enables API-based research on large pre-trained models and guards against misuse;
- allows researchers to use their NAIRR compute budget to do research on models accessed through an API; and
- explores ways to incentivize technology companies, academic researchers, and government agencies to provide structured access to large pre-trained models through the API.
Signs of a trend
We predict that an increasing portion of important AI research and development will make use of large pre-trained models that are accessible only through APIs. In this paradigm, pre-trained models would play a central role in the AI ecosystem. A large portion of SOTA models would be developed by fine-tuning(2) and otherwise adapting these models to particular tasks. Commercial considerations and misuse concerns would also frequently prevent developers from granting others access to their pre-trained models, except through APIs. Though we are still far from being in this paradigm, there are some early indications of a trend.
Particularly in the domain of natural language processing, academic research is beginning to build upon pre-trained models such as T5, BERT, and GPT-3. At one of the leading natural language processing conferences in 2021, EMNLP, a number of papers were published that investigated and evaluated existing pre-trained models. Some of the most relevant models are accessible only or primarily through APIs. The OpenAI API for GPT-3, announced in June 2020, has been used in dozens of research papers, for example investigating the model’s bias, its capabilities, and its potential to accelerate AI research by automating data annotation. Furthermore, Hugging Face’s API interface has been used to investigate COVID-19 misinformation and to design a Turing test benchmark for language models.
At the same time, in the commercial domain, applications of AI increasingly rely on pre-trained models that are accessed through APIs. Amazon Web Services, Microsoft Azure, Google Cloud, and other cloud providers now offer their customers access to pre-trained AI systems for visual recognition, natural language processing (NLP), speech-to-text, and more. OpenAI reported that its API for its pre-trained language model GPT-3 generated an average of 4.5 billion words per day as of March 2021, primarily for commercial applications.
Five underlying factors in the AI field explain why we might expect a trend towards academic research that relies on large pre-trained models that are only accessible through APIs:
- Training SOTA models from scratch requires large amounts of compute, precluding access for actors with smaller budgets. For instance, PaLM – a new SOTA NLP model from Google Research – is estimated to have cost between $9 and $17M to train. The training compute cost of developing the next SOTA NLP model will likely be even greater.
- In comparison, conducting research on pre-trained models typically requires small compute budgets. For instance, we estimate that a recent paper investigating anti-muslim bias in GPT-3 likely required less than $100 of compute(3). Developing new SOTA models by fine-tuning or otherwise adapting “foundation models” will also typically be dramatically cheaper than developing these models from scratch.
- The developers of large pre-trained models are likely to have strong incentives not to distribute these models to others, as this would make it both more difficult to monetize the models and more difficult to prevent misuse.
- Given the right infrastructure, it is significantly easier for researchers to use a pre-trained model that is accessed through an API than it is for them to implement the model themselves. Implementing large models, even for research purposes, can require significant engineering talent, expertise, and computing infrastructure. Academics and students often lack these resources.
- Academics may increasingly aim their research at understanding and scrutinizing models, as this is important scientific work and plays to academia’s comparative advantage.
We discuss these factors in detail below, in an appendix to this post.
How the NAIRR could provide access to pre-trained models
We offer a sketch of how the NAIRR could provide access to pre-trained models in addition to data and compute, illustrated in the figure below. First, it would create a platform for hosting and accessing pre-trained models via an API. The platform should be flexible enough to allow researchers to run a wide range of experiments on a range of models. It should be capable of supporting fine-tuning, interpretability research, and easy comparison of outputs from multiple models. The API should allow researchers to interface with both models hosted by the NAIRR itself and models hosted by other developers, who may often prefer to retain greater control over their models.
Second, researchers would be allowed to use their NAIRR compute budgets to run inferences on the models. We recommend that researchers be allowed to use their budgets for this purpose even if the model is hosted by an organization other than the NAIRR.
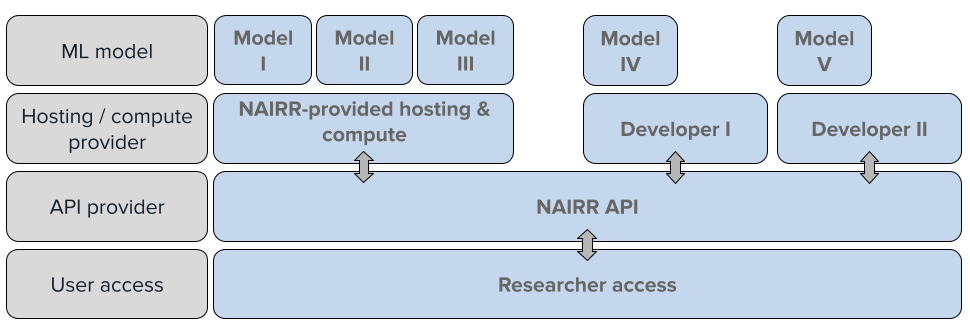
The biggest challenge will likely be securing access to pre-trained models from developers across industry, academia, and government. In some cases, developers might be motivated to provide access by a desire to contribute to scientific progress, the prospect of external actors finding issues and ways to improve the model, or a belief that it might improve the organization’s reputation. The NAIRR could also create an expectation that models trained using NAIRR compute should be accessible through the platform. Access to particularly high-stakes government models in need of outside scrutiny could also potentially be mandated. Additionally, the NAIRR could consider incentivizing government agencies to provide API access to some of their more impactful models in exchange for access to compute resources or data (similar to a Stanford HAI proposal regarding data access).
Encouraging private actors to make their models accessible through the platform may be especially difficult. In some cases, companies may provide model access as a means to build trust with their consumers. They may recognize that the public will be far more trusting of claims concerning the safety, fairness, or positive impacts of their AI systems if these claims are vetted by outside researchers. For example, Facebook and Twitter have recently created APIs that allow outside researchers to scrutinize company data in a privacy-preserving manner. Further, the NAIRR could consider offering compensation to developers for making their models available via the API. Developers may also be particularly concerned about risks to intellectual property, something that can be assuaged by the NAIRR upholding high cybersecurity standards.
Crucially, the API should also be designed to thwart model misuse, while still ensuring easy access for research use. Multi-purpose models trained with NAIRR resources could be used maliciously, for instance by criminals, propagators of misinformation, or autocratic governments around the world. Large language models could, for example, significantly reduce the cost of large-scale misinformation campaigns. The NAIRR should take measures to avoid models trained with publicly funded compute being put to such uses. Misuse could be reduced by introducing a tiered access approach, as suggested in the Stanford HAI report for datasets hosted on the NAIRR. For instance, researchers might get easy access to most models but need to apply for access to models with high misuse potential. Further restrictions could then be placed on the queries or modifications that researchers are allowed to make to certain models. In addition, API usage should be monitored for suspicious activity (e.g. the generation of large amounts of political content).
Helping academic researchers share their models
An appropriately designed API could also solve a challenge the NAIRR will face as it provides compute and data for the training of large-scale models: academic researchers will likely want to share and build on models developed with NAIRR resources. At the same time, open-sourcing the models may come with the risk of misuse in some cases. By building an API and agreeing to host models itself, the NAIRR can address this problem: it can make it easy for researchers to share their models in a way that is responsive to misuse concerns.
Academics are significantly more likely to voluntarily make their models available via the API than private developers of SOTA models with a profit motive. As such, the NAIRR could start by focusing on providing infrastructure for academic researchers to share their models with each other, thereby building a proof-of-concept, and later introducing additional measures to secure access to models produced in industry and across government.
Conclusion
By building API infrastructure to support access to large pre-trained models, the NAIRR could produce a number of benefits. First, it could help academics to scrutinize and understand the most capable and socially impactful AI models. Second, it could cost-effectively grant researchers and students the ability to work on frontier models. Third, it could help researchers to share and build upon each other’s models while also avoiding risks of misuse. Concretely, we recommend that the NAIRR:
- provides infrastructure that enables API-based research on large pre-trained models and guards against misuse;
- allows researchers to use their NAIRR compute budget to do research on models accessed through an API; and
- explores ways to incentivize technology companies, academic researchers, and government agencies to provide structured access to large pre-trained models through the API.
Appendix: Five factors underlying our prediction that pre-trained models accessed via APIs will become increasingly central to academic AI research
Training SOTA models requires large amounts of compute
Training a SOTA model often requires large amounts of computational resources. Since 2012, the computational resources for training SOTA models have been doubling every 5.7 months.
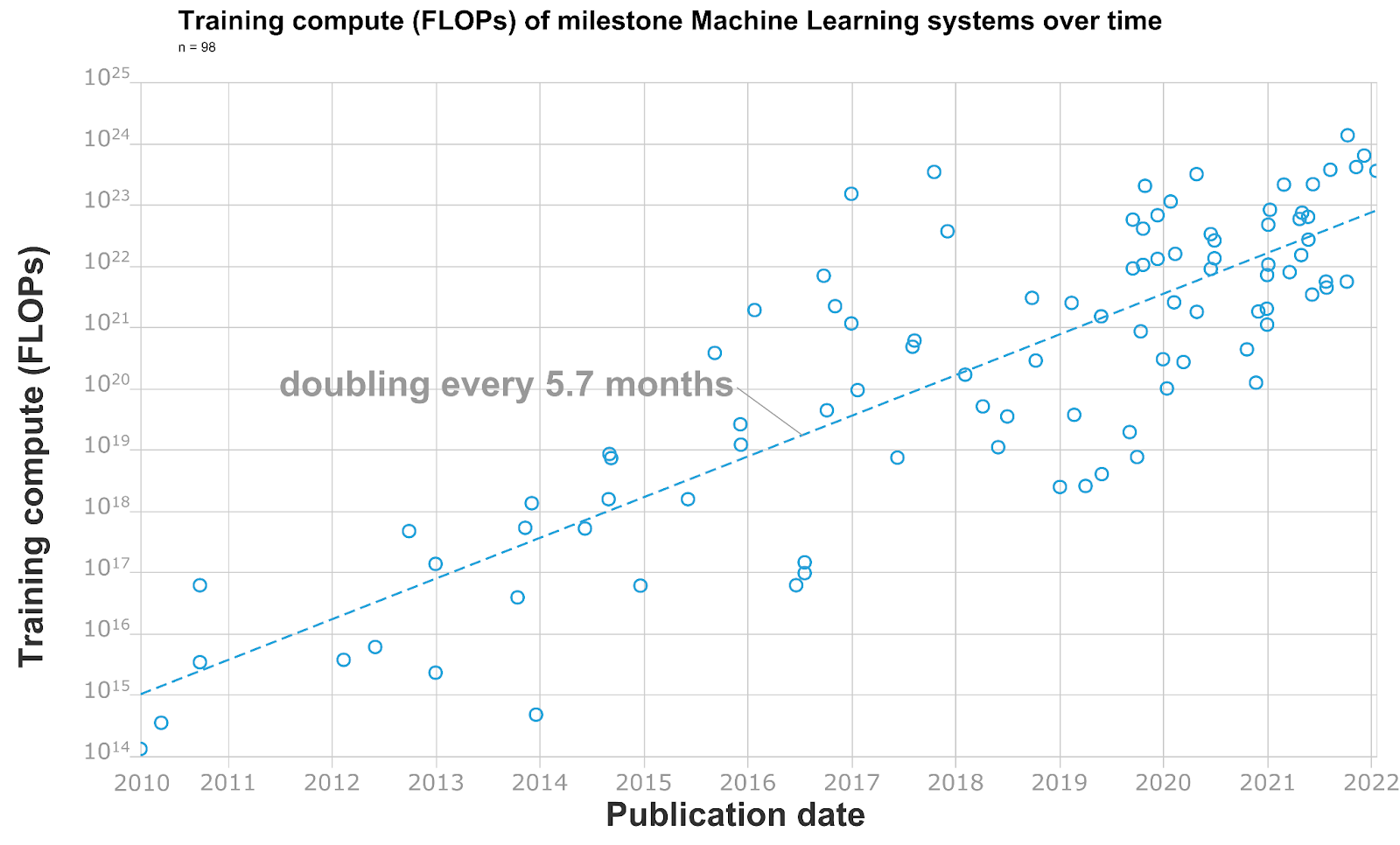
The final training run of AlphaGo Zero in 2017 is estimated to have cost $35M(4). GPT-3, a SOTA NLP model developed in 2020 that is accessible via an API, has been estimated to have cost around $4.6M to train.2 Gopher – a recent frontier NLP model developed in 2021 by DeepMind – already doubled the compute requirements, costing around $9.2M.(5) PaLM, a new SOTA NLP model from Google Research, is estimated to have cost between $9 and $17M to train. The training compute cost of developing the next SOTA NLP model will likely be even greater.
Research on pre-trained models requires small compute budgets
Second, research on pre-trained models is much less compute-intensive in comparison. For example, if one would have spent the computational resources required to train GPT-3 on inference rather than training, one could have produced up to 56 billion words — that’s 14-times the number of words of the English Wikipedia.(6) We estimate that a recent paper investigating anti-muslim bias in GPT-3 likely required less than $100 of compute.(7) Fine-tuning of pre-trained models also appears very low cost, at least in natural language processing. OpenAI charges $120 for fine-tuning GPT-3 on 1 million tokens, which is more than the company used in a recent paper to fine-tune GPT-3 to avoid toxic outputs.
Through access to pre-trained models, many more people can cheaply access high-end AI capabilities, including people from domains outside AI and AI researchers who lack access to large amounts of compute and teams of engineers. To illustrate the low cost, we estimate that every US AI PhD student could be provided with five times the compute required to produce a paper on biases in large language models for the cost of training one Gopher-sized model (around $9.5M).(8)
Relative to open-sourcing, model access via API reduces the chance of misuse and supports model monetization
Third, the ability to provide structured access may incentivize producers to make their models available via API rather than open-sourcing them. Using an API, developers can allow access to their models while curtailing misuse and enabling monetization. AI models can be used for ill, for instance through disinformation, surveillance, or hacking. Large language models can also reveal privacy-infringing information, not intended by their developers. By only providing access to the model via an API, a developer can put in place checks, tripwires, and other forms of monitoring and enforce terms of service to avoid the model’s inappropriate use. They can also introduce restrictions on the inputs that can be sent to the models and update these restrictions over time, for instance to close loopholes or address newly discovered forms of misuse.
Although open-source models typically provide researchers with a greater deal of flexibility than API access, this discrepancy can be reduced. Access via API does not need to be limited to only running inference on a given input; API functionality can go further. Fundamental tasks, such as fine-tuning the model, can and should be enabled to offer a wide variety of research. For example, OpenAI recently allowed the fine-tuning of GPT-3 via API access, letting users customize this language model to a desired task. Google’s Vision API and Cohere’s language model also offer customization via fine-tuning. In the future, more functionality could be introduced to ensure that research via API is only minimally restrictive. For instance, it is possible to allow external researchers to remotely analyze a model’s inner workings without giving away its weights.
Companies are also likely to increasingly offer access to their most powerful models via API, rather than by open-sourcing them, as doing so provides them with the opportunity to monetize their models. Examples include OpenAI, Cohere, Amazon Web Services (AWS), and Google Cloud which allow access to their models solely via an API, for a fee.
Given the right infrastructure, it is significantly easier for researchers to use a pre-trained model that is accessed through an API than it is for them to implement the model themselves
Doing AI research and building products using pre-trained models accessed via API has some advantages compared to implementing the model oneself. Implementing large models, even for research purposes, can require significant engineering talent, expertise, and computing infrastructure. Academics and students often lack these resources and so might benefit from API access.
It is becoming increasingly inefficient to do “full-stack” AI research. AI research is seeing an increasing division of labor between machine learning engineers and researchers, with the former group specializing in how to efficiently run and deploy large-scale models. This is a natural development: as a field matures, specialization tends to increase. For instance, AI researchers virtually always rely on software libraries developed by others, such as Tensorflow and Pytorch, rather than starting from scratch. Similarly, an increasing portion of tomorrow’s AI research could be done largely by building on top of pre-trained models created by others.
Scrutinizing large-scale models may be increasingly important research and plays to the comparative advantage of academic researchers
Lastly, academic researchers may be increasingly drawn to research aimed at scrutinizing and understanding large-scale AI models, as this could constitute some of the most important and interesting research of the next decade and academics could be particularly well-suited to conduct it.
As AI systems become more sophisticated and integrated into our economy, there’s a risk that these models become doubly opaque to society: Firstly, the workings of the models themselves may be opaque. Secondly, the model developer might not reveal what they know about its workings to the wider world. Such opacity undermines our ability to understand the impacts of these models and what can be done to improve their effects. As a result, research aimed at understanding and auditing large models could become increasingly valued and respected.
Academic researchers are also particularly well-suited to conducting this kind of research. Many are drawn to academia, rather than industry, because they are motivated by a desire for fundamental understanding (e.g. how and why AI systems work) and care relatively less about building systems that achieve impressive results. On average, researchers who decide to stay in academia (and forgo much higher salaries) are also more likely to be concerned about the profit incentives and possible negative social impacts of private labs. This suggests that academics could find scrutinizing private labs’ models appealing. On the other hand, in addition to access to large amounts of compute and the ability to implement large models, there are a number of factors that place private labs at an advantage with regard to developing large models. Private labs have the ability to offer higher salaries, vast datasets, the infrastructure necessary to deploy models in the real world, and strong financial incentives to develop models at the frontier, as these models can be integrated into billion-dollar products like search and news feeds.
Some early examples are already beginning to emerge, which illustrate how this division of responsibilities could work in practice. For instance, Facebook and Twitter recently opened up APIs that give researchers and academics access to data of user interactions with their platforms in a safe, privacy-preserving environment.
IVR Business Owner
Job title: IVR Business Owner
Company: SSE
Job description: | Full Time | options available What is the Role? Our IVR Business Owner is tasked with overseeing the IVR system. You’ll… and customer experience objectives. – evaluate and prioritise change requests based on business impact, customer feedback…
Expected salary:
Location: Dublin – Belfast
Job date: Thu, 16 May 2024 07:52:59 GMT
Apply for the job now!
Announcing the GovAI Policy Team
The AI governance space needs more rigorous work on what influential actors (e.g. governments and AI labs) should do in the next few years to prepare the world for advanced AI.
We’re setting up a Policy Team at the Centre for the Governance of AI (GovAI) to help address this gap. The team will primarily focus on AI policy development from a long-run perspective. It will also spend some time on advising and advocating for recommendations, though we expect to lean heavily on other actors for that. Our work will be most relevant for the governments of the US, UK, and EU, as well as AI labs.
We plan to focus on a handful of bets at a time. Initially, we are likely to pursue:
- Compute governance: Is compute a particularly useful governance node for AI? If so, how can this tool be used to meet various AI governance goals? Potential goals for compute governance include monitoring capabilities, restricting access to capabilities, and identifying high-risk systems such that they can be put to significant scrutiny.
- Corporate governance: What kinds of corporate governance measures should frontier labs adopt? Questions include: What can we learn from other industries to improve risk management practices? How can the board of directors most effectively oversee management? How should ethics boards be designed?
- AI regulation: What present-day AI regulation would be most helpful for managing risks from advanced AI systems? Example questions include: Should foundation models be a regulatory target? What features of AI systems should be mandated by AI regulation? How can we help create more adaptive and expert regulatory ecosystems?
We’ll try several approaches to AI policy development, such as:
- Back-chaining from desirable outcomes to concrete policy recommendations (e.g. how can we increase the chance there are effective international treaties on AI in the future?);
- Considering what should be done today to prepare for some particular event (e.g. the US government makes an Apollo Program-level investment in AI);
- Articulating and evaluating intermediate policy goals (e.g. “ensure the world’s most powerful AI models receive external scrutiny by experts without causing diffusion of capabilities”);
- Analyzing what can and should be done with specific governance levers (e.g. the three bets outlined above);
- Evaluating existing policy recommendations (e.g. increasing high-skilled immigration to the US and UK);
- Providing concrete advice to decision-makers (e.g. providing input on the design of the US National AI Research Resource).
Over time, we plan to evaluate which bets and approaches are most fruitful and refine our focus accordingly.
The team currently consists of Jonas Schuett (specialisation: corporate governance), Lennart Heim (specialisation: compute governance), and myself (Markus Anderljung, team lead). We’ll also collaborate with the rest of GovAI and people at other organisations.
We’re looking to grow the team. We will be hiring Research Scholars on the Policy Track on a regular basis. We’re also planning to work with people in the GovAI 3-month Fellowship and are likely to open applications for Research Fellows in the near future (you can submit expressions of interest now). We’re happy for new staff to work out of Oxford (where most of GovAI is based), the Bay Area (where I am based), or remotely.
If you’d like to learn more, feel free to leave a comment below or reach out to me at
ma***************@go********.ai
.