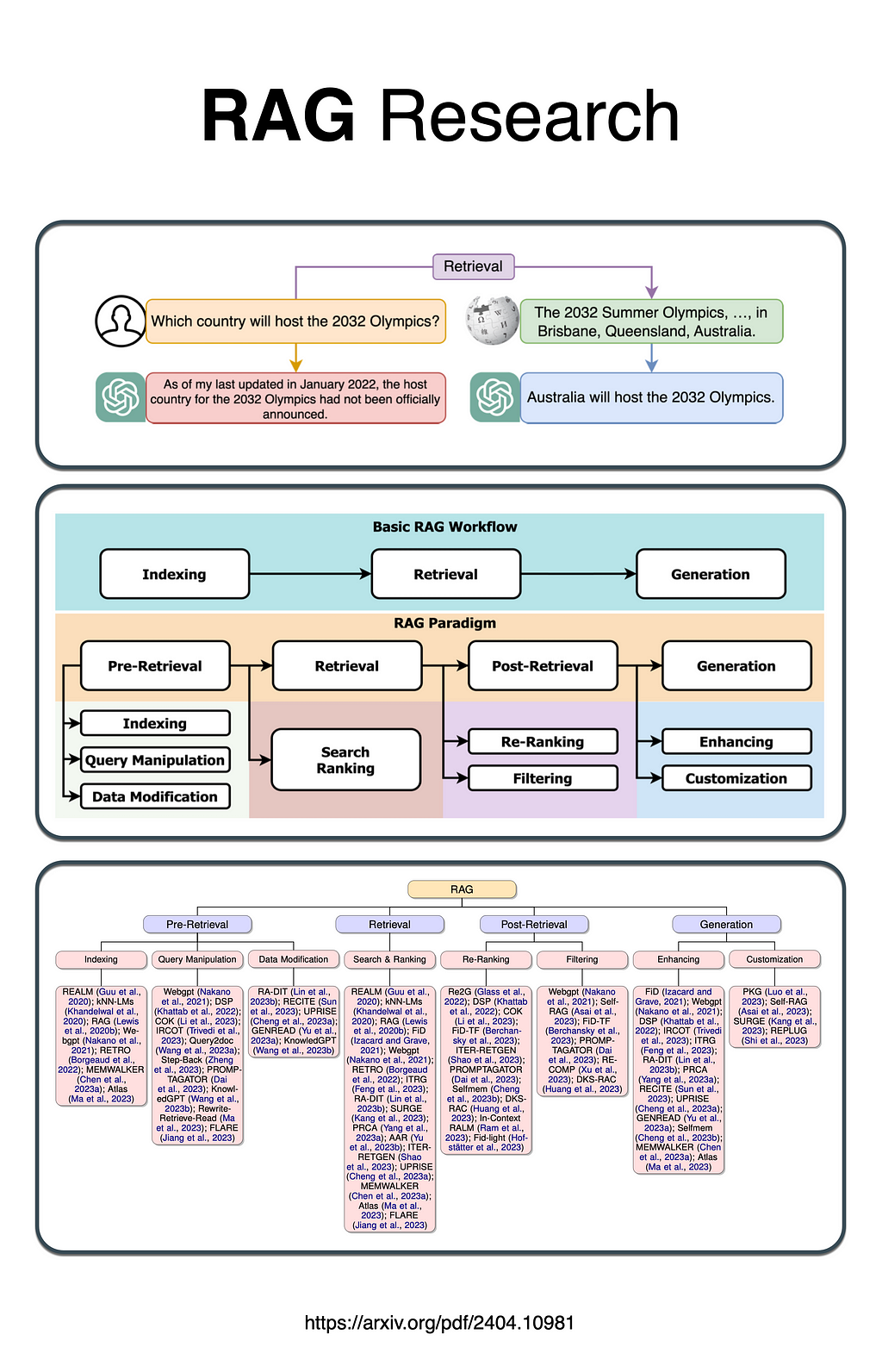
RAG Survey & Available Research
A Survey on Retrieval-Augmented Text Generation for Large Languageย Models
Recap Onย RAG
Retrieval-Augmented Generation (RAG) combines retrieval methods with In-Context Learning (ICL) & Natural Language Generation (NLG) to overcome the static knowledge limitations of large language models (LLMs) by integrating dynamic external information.
This approach primarily targets the text domain and offers a cost-effective solution to mitigate the generation of plausible but incorrect responses by LLMs, thus improving their accuracy and reliability through the use of real-world data.
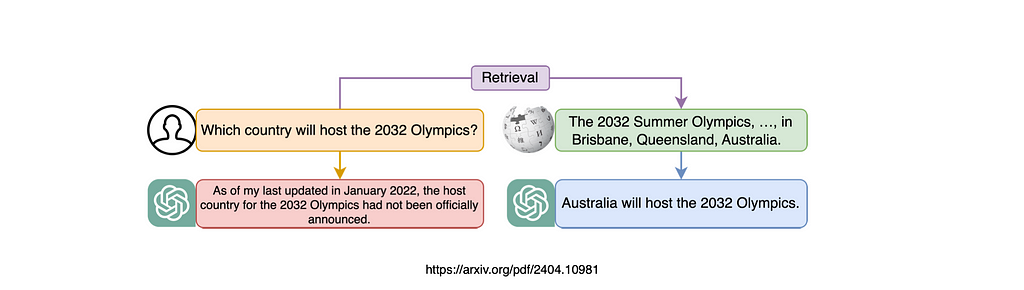
๐ฅ๐๐ ๐ถ๐ ๐ฐ๐ฎ๐๐ฒ๐ด๐ผ๐ฟ๐ถ๐๐ฒ๐ฑ ๐ถ๐ป๐๐ผ ๐ณ๐ผ๐๐ฟ ๐ธ๐ฒ๐ ๐๐๐ฎ๐ด๐ฒ๐:
๐ฃ๐ฟ๐ฒ-๐ฟ๐ฒ๐๐ฟ๐ถ๐ฒ๐๐ฎ๐น,
๐ฅ๐ฒ๐๐ฟ๐ถ๐ฒ๐๐ฎ๐น,
๐ฃ๐ผ๐๐-๐ฟ๐ฒ๐๐ฟ๐ถ๐ฒ๐๐ฎ๐น, &
๐๐ฒ๐ป๐ฒ๐ฟ๐ฎ๐๐ถ๐ผ๐ป.
It also introduces evaluation methods for RAG, addressing the challenges faced and suggesting future research directions. By offering a structured framework and categorisation, the study aims to consolidate existing research on RAG, clarify its technological foundations, and emphasise its potential to expand the adaptability and applications ofย LLMs.
The paper highlights how RAG can dynamically integrate up-to-date information to enhance the performance of LLMs, making them more reliable and effective in generating accurate responses, thereby broadening their practical uses in variousย domains.
The image below shows a basic RAG workflow, but also the sub-components liked to the four RAGย steps.
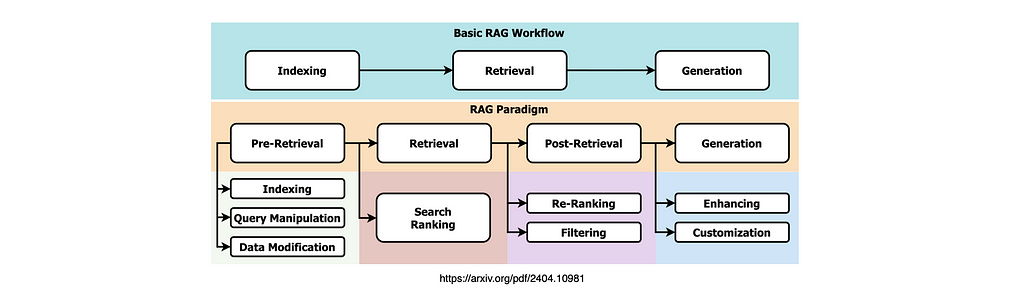
The image below contains a list of all the existing research for each of RAG components.
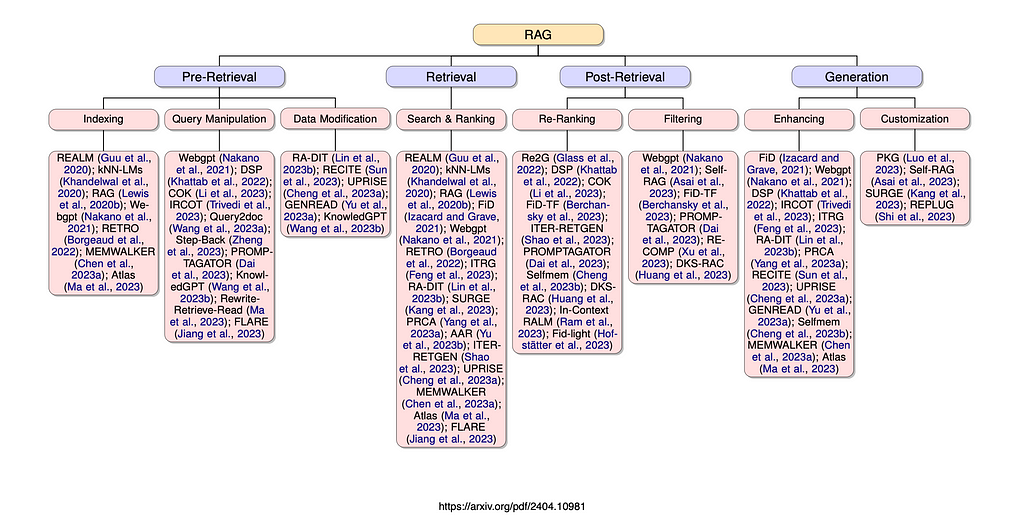
โญ๏ธ Follow me on LinkedIn for updates on Large Language Modelsย โญ๏ธ

Iโm currently the Chief Evangelist @ Kore AI. I explore & write about all things at the intersection of AI & language; ranging from LLMs, Chatbots, Voicebots, Development Frameworks, Data-Centric latent spaces &ย more.

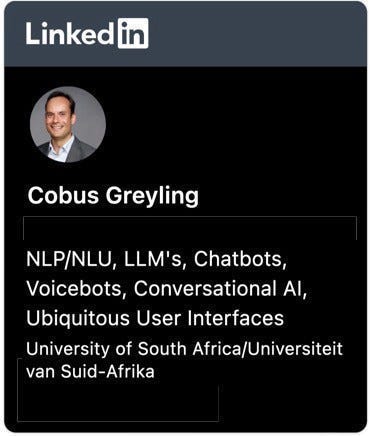
